Aggregated Foot Traffic Datasets
Validate your next business decision with our machine learning-powered foot traffic datasets for the U.S.
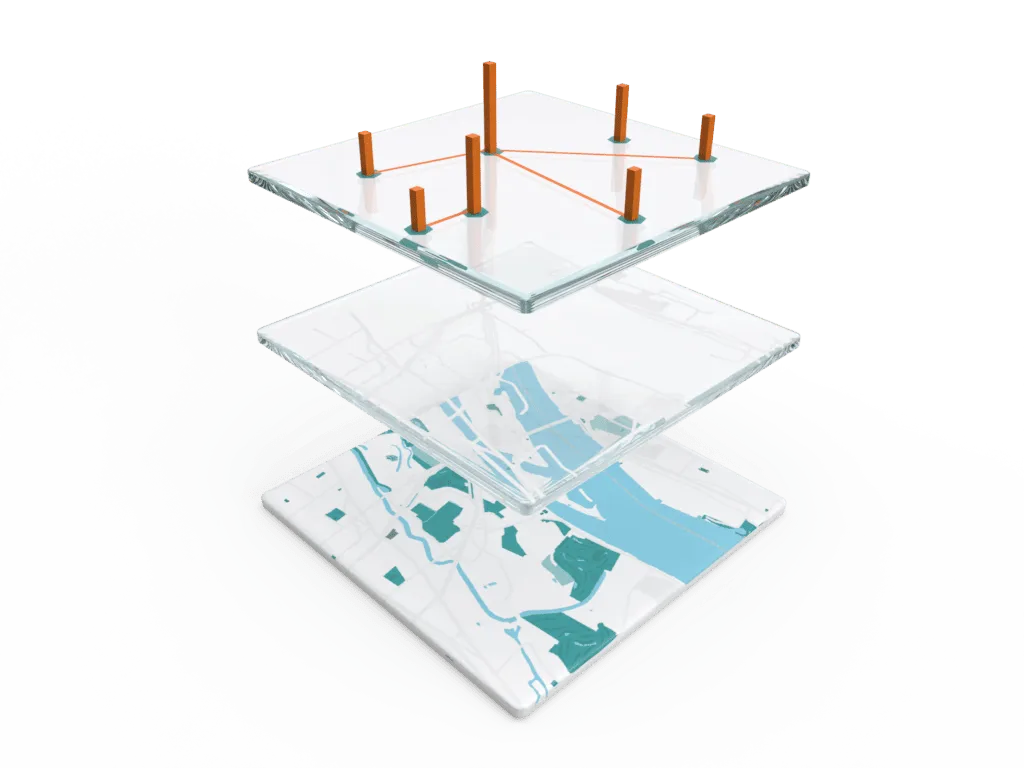
Validate your next business decision with our machine learning-powered foot traffic datasets for the U.S.
Our aggregated foot traffic datasets can help you understand foot traffic activity in an area or location of interest, as well as the origins, cross visits, and demographic profiles of your visitors. With machine-learning powered insights, you can make more informed decisions for site selection or deselection, boost your competitive advantage, and so much more.
Learn where, when, and which kinds of customers visit your competitors’ locations instead of yours (and vice versa).
Measure the impact of retail optimizations, new products, and promotions.
Identify gaps in the local marketplace and other opportunities to expand your portfolio.
Understand how individual stores within a given brand are performing to selectively close or promote them.
Validate potential opportunities and reduce investment risk
Use foot traffic data to predict revenue and optimize your logistics and supply chain.
Unacast provides foot traffic, trade area, cross visitation and demographic data to help you solve your biggest business problems.
Our foot traffic data helps you understand visitation at a specific site or area – how many people visit, types of visitors, and traits of the visit.
Make better decisions by understanding the home and work locations of your visitors.
Discover other shops and locations your customers visit.
Determine what types of people are visiting a location.
Unlike typical aggregated products that rely solely on aggregating the underlying GPS device-level supply, our machine learning model is more robust and less dependent on GPS data fluctuations because it is based on a multitude of different data sources.
Our models begin with privacy-friendly first party location data. That data is then combined with contextual data including demographics, industry trends, venue attributes, historical data, and more.
We train our machine learning models to learn the relationship between different data sources and our target mobility insights.
In total, the model comprises more than 120 features to train those relationships based on our long history of high-quality location data.
Our machine learning-powered data is then curated into easy-to-work-with foot traffic datasets.
A common issue with using ML models is that they give you a prediction without any understanding of whether it is right or wrong. When validated against ground truth data, Unacast’s models recorded an R-squared value of up to .93, widely considered to be best-in-class.