Introduction
Welcome to Unacast’s Comprehensive Retail Analytics Knowledge Hub. This meticulously curated repository gleans insights from across the industry and assembles them in one central location for your convenience. Begin your exploration with the foundational aspects outlined on this page, and we'll dive into specific facets of Retail Analytics, including its varied applications, pioneering trends, vital tools and technologies, real-world examples, and much more. Whether you're a seasoned professional or just starting in the realm of retail, this hub is designed to empower your strategies with data-driven wisdom.
What is Retail Analytics?
At its core, Retail Analytics can be defined as the process of providing analytical data on inventory levels, sales, supply chain movement, customer demand, and more, that assists merchants in making informed decisions. Essentially, it's where data science and retail converge to optimize every facet of the retail process. Top retail analytics companies, as well as location analytics software exist to help you leverage key location data insights to improve your retail business.
The retail space generates a plethora of data daily: from sales receipts and loyalty cards to e-commerce metrics and social media feedback. Retail Analytics harnesses this data, processes it, and then translates it into actionable insights. For instance, by analyzing sales data, a retailer can ascertain which products are the top performers and which ones are lagging, thus aiding in inventory management.
A pivotal component of Retail Analytics is its dual focus: both on the back end (supply chain, stock levels, procurement) and the front end (customer behavior, foot traffic data, sales patterns, and trends). By amalgamating these two vast pools of information, Retail Analytics provides a holistic view of the retail environment, allowing businesses to be proactive rather than reactive.
Benefits of Retail Analytics
The immense power of Retail Analytics lies in its potential to offer myriad benefits to businesses, regardless of their scale or domain. Here are some of the most salient advantages:
1. Enhanced Customer Experience: By understanding customer behavior and preferences, retailers can personalize the shopping experience, leading to increased satisfaction and loyalty. For instance, analyzing purchase history can aid in tailoring marketing campaigns to specific demographics.
2. Inventory Management and Optimization: No more overstocking or stockouts. With insights into which products are selling and which are not, retailers can manage their inventory more effectively, minimizing costs and maximizing sales.
3. Price Optimization: Retailers can tweak prices based on demand, competition, and other external factors. Dynamic pricing, facilitated by analytics, ensures that the price is always right to maximize profit and sales.
4. Sales Forecasting: With the power of predictive analytics, businesses can forecast future sales with higher accuracy, aiding in planning and strategy formulation.
5. Identifying Market Shifts: Spotting emerging trends before they become mainstream gives retailers an edge. Retail Analytics provides insights into changing consumer preferences, allowing businesses to pivot or adapt swiftly.
In summation, Retail Analytics not just boosts the bottom line but also refines the entire retail process, fostering efficiency, adaptability, and foresight.
Using Retail Analytics to Identify Locations for Expansion
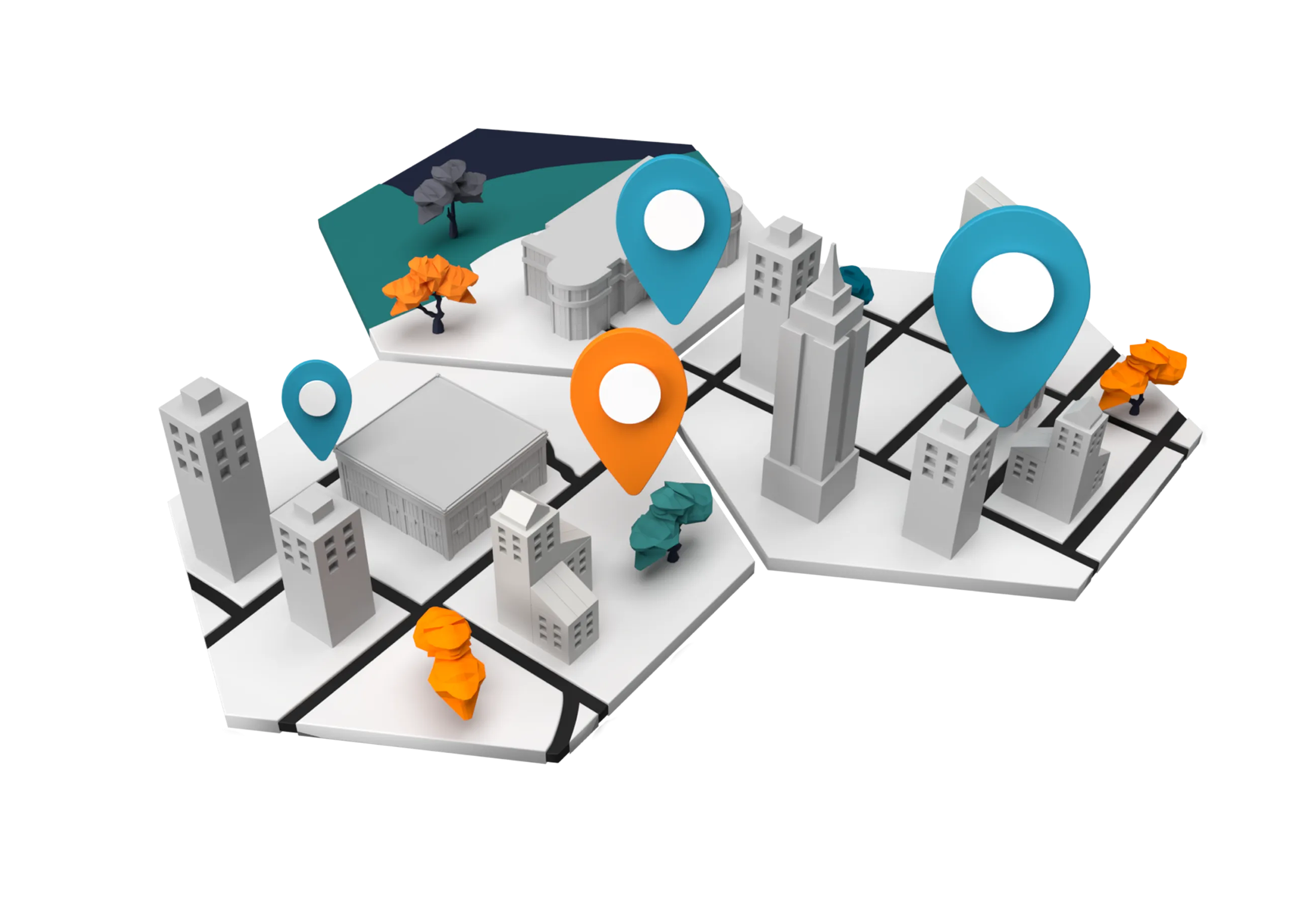
Retailers increasingly leverage retail analytics to strategically select new store locations based on foot traffic and other pertinent data. Here's how they do it:
1. Historical Foot Traffic Analysis: By studying retail foot traffic data and patterns in various areas, retailers can identify locations that consistently attract a high volume of pedestrians, suggesting potential for robust store traffic.
2. Demographic and Socioeconomic Data Integration: Retail analytics can merge foot traffic data with demographic and socioeconomic information. This ensures that the foot traffic in a given location aligns with the retailer's target audience, optimizing the potential for sales.
3. Competitor Analysis: Analytics tools can evaluate the presence and performance of competitors in potential locations. Retailers can then decide to tap into underserved markets or strategically position themselves amidst competition.
4. Event-Based Traffic Analysis: Retailers can assess how local events, festivals, or other gatherings influence foot traffic. Locations with regular events may offer additional sales opportunities.
5. Predictive Modeling: Advanced analytics can forecast potential foot traffic and sales based on a combination of factors, including local trends, population growth, and planned infrastructure or commercial developments.
6. Real-Time Data Analysis: Using real-time data from mobile devices and connected technologies, retailers can get immediate insights into current foot traffic patterns, providing a dynamic view that complements historical data.
By leveraging these data points, retail analytics equips retailers with a holistic view of potential store locations, ensuring their new outlets are strategically positioned for maximum success.
Types of Businesses that Use Retail Analytics & How They Use It
Retail Analytics isn’t confined to a singular type of business; its application stretches across a spectrum of retail entities, each harnessing data in distinctive ways:
1. Brick-and-Mortar Stores: Traditional retail outlets employ analytics to optimize the physical shopping experience. By analyzing footfall data, these businesses can gauge peak shopping hours, guiding staffing decisions. Furthermore, store layout optimization, derived from tracking customer movement, ensures high-margin products are placed in high-traffic areas.
2. E-commerce Platforms: Digital retailers have a vast reservoir of data at their fingertips. Website traffic analysis helps identify the most visited pages, while cart abandonment insights pinpoint potential friction points in the buying process. Moreover, analytics empower e-retailers to offer personalized product recommendations, elevating the shopping experience.
3. Omnichannel Retailers: These are businesses operating both offline and online. Retail Analytics allows them to create a unified customer experience. By analyzing cross-platform sales data, they can devise strategies to promote in-store pickups or encourage online sales through exclusive web promotions.
Necessary Capabilities for Retail Analytics
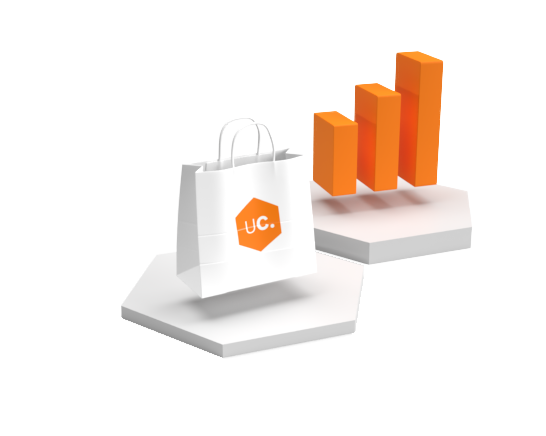
To harness the full potential of Retail Analytics, businesses need to ensure they are equipped with certain capabilities:
1. Technological Infrastructure: This serves as the bedrock for any analytical endeavor. Modern Point of Sale (POS) Systems and Customer Relationship Management (CRM) Software are essential for capturing and organizing vast amounts of data.
2. Analytical Skillset: Merely collecting data isn’t sufficient. You need a team of skilled data scientists and analysts who can transform this data into actionable insights. These professionals should have a sound understanding of retail Key Performance Indicators (KPIs) and the nuances of the retail landscape.
3. Data Governance and Privacy: Ethical data collection is paramount. Retailers must ensure compliance with privacy laws and regulations, guaranteeing that consumer data is collected transparently and stored securely.
Challenges in Retail Analytics and Overcoming Them
Despite its numerous advantages, implementing Retail Analytics isn’t devoid of challenges:
Data Silos and Integration Issues: Often, data is stored in isolated systems, making integration cumbersome. Overcoming this requires an integrated IT infrastructure where all data sources communicate seamlessly.
Real-Time Analysis Constraints: The retail environment is dynamic, and data can become obsolete quickly. Real-time analysis requires advanced analytical tools and a robust IT infrastructure.
Training and Change Management: Introducing new analytical tools can be disruptive. It’s crucial to have a comprehensive training program in place for staff to understand and utilize these tools effectively.
Ensuring Data Accuracy and Quality: Garbage in, garbage out. Poor data quality can lead to flawed insights. Retailers must implement rigorous data validation and cleaning protocols to ensure the integrity of their analyses.
Addressing these challenges head-on, with a mix of technology, training, and robust processes, ensures that Retail Analytics becomes a transformative force within the retail landscape.
Future Trends in Retail Analytics
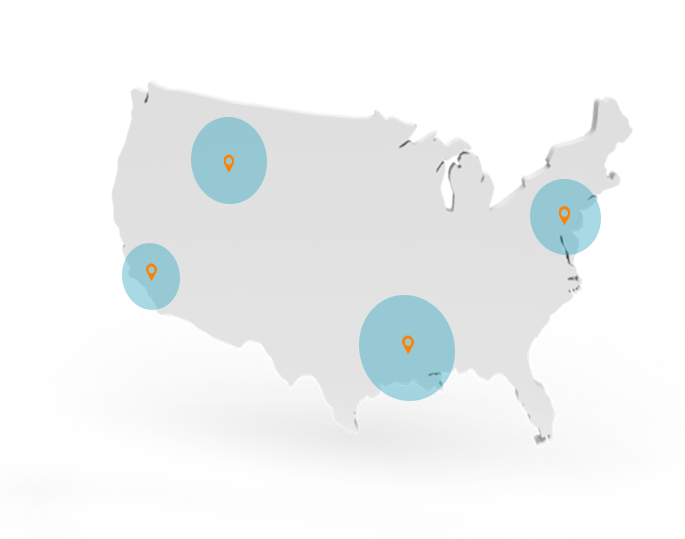
As technology continues to evolve at a breakneck pace, the realm of Retail Analytics is poised for a series of transformative shifts. Here are the trends to watch:
1. Integration of AI and Machine Learning: Artificial Intelligence (AI) and Machine Learning (ML) are not just buzzwords but powerful tools shaping the future of analytics. With ML algorithms, retailers can predict customer behavior with unprecedented precision, tailor marketing strategies in real time, and optimize inventory management.
2. The Rise of Predictive Analytics: Moving beyond reactive decision-making, retailers will increasingly rely on predictive models to forecast trends, sales, and inventory needs. This proactive approach will provide businesses with a significant competitive advantage.
3. Virtual Reality (VR) and Augmented Reality (AR) in Retail Analysis: As AR and VR technologies become mainstream, their application in retail will grow. From virtual trial rooms to AR-based store navigation, the combination of immersive technology and analytics will redefine the shopping experience.
4. The Role of Big Data: The amount of data being generated is increasing exponentially. Big Data tools and technologies will be paramount in storing, processing, and extracting insights from this vast reservoir of information.
5. Personalization at Scale: Retailers will harness analytics to provide a hyper-personalized shopping experience, offering product recommendations, discounts, and marketing messages tailored to individual customer preferences and behaviors.
Conclusion
Retail Analytics, once a peripheral element of the retail industry, has cemented its position as a central pillar of modern retail strategy. As we've journeyed through its various facets, from its intrinsic benefits to its future trajectory, one truth emerges: Retail Analytics is no longer a luxury but a necessity for businesses aiming for longevity and relevance in an increasingly data-driven world. Embracing the methodologies and tools of analytics equips retailers to navigate the complex and dynamic landscape of modern commerce with agility, foresight, and precision. As we stand at the cusp of another retail revolution, fueled by technological innovation, Retail Analytics emerges as the compass guiding businesses towards unprecedented growth, efficiency, and customer satisfaction. Those ready to invest in, and understand, this domain will undoubtedly be the vanguards of the next era of retail excellence.
Frequently Asked Questions about Retail Analytics
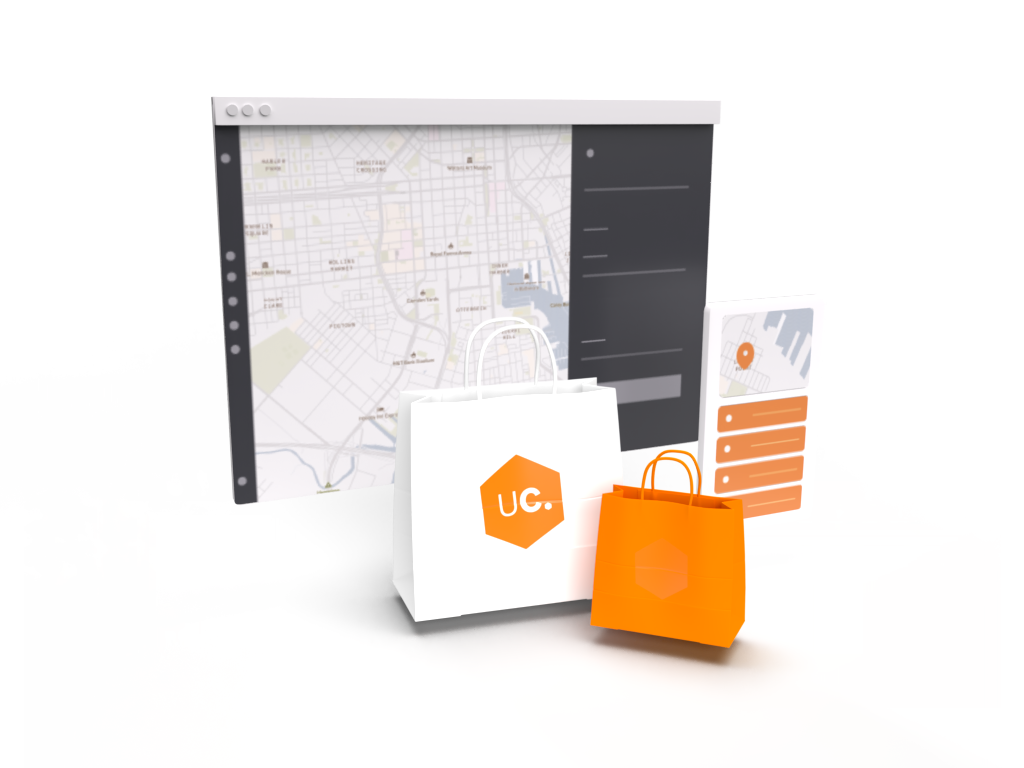
What is an example of data analysis in retail?
One prominent example of data analysis in retail is the "Market Basket Analysis." This analytical technique seeks to understand the combinations of products that customers frequently buy together. For instance, through market basket analysis, a retailer might discover that customers who buy diapers often purchase baby wipes and baby lotion during the same shopping trip. Recognizing such patterns, a store could strategically place these items near each other or bundle them in promotions, thereby enhancing sales and customer convenience.
Another application of this analysis is in online retail. E-commerce platforms use market basket analysis to suggest products to users. If you've ever seen a "Customers who bought this item also bought..." recommendation, that's market basket analysis in action.
What are the types of retail data analytics?
Retail data analytics can be broadly classified into four types:
Descriptive Analytics: This is the most fundamental form. It answers the question, "What happened?" By analyzing past data, it provides insights into sales trends, customer behaviors, and inventory levels.
Diagnostic Analytics: Here, the focus is on understanding why something happened. For instance, if a retailer noticed a sudden spike in sales, diagnostic analytics would delve into the reasons, whether it was a specific marketing campaign, a holiday season, or a trending product.
Predictive Analytics: As the name suggests, this focuses on predicting future outcomes based on historical data. For example, a retailer could predict which products are likely to be bestsellers in the upcoming season or forecast inventory needs.
Prescriptive Analytics: This is a more advanced form, offering recommendations on what actions to take to achieve desired outcomes. If predictive analytics forecasts a high demand for a product, prescriptive analytics might suggest increasing stock levels or launching a complementary marketing campaign.
How do I use data analytics in retail?
Implementing data analytics in retail involves a structured approach:
Data Collection: Begin by gathering data from various sources – POS systems, e-commerce platforms, loyalty programs, and customer feedback.
Data Cleaning and Integration: Ensure the data is accurate and consistent. Integrate data from different sources to create a comprehensive dataset.
Choose the Right Tools: Invest in analytical tools and software that cater to your specific retail needs. Platforms like Tableau, Microsoft Power BI, and Google Analytics can be beneficial.
Analyze and Interpret: With tools in place, analyze the data. Use the types of retail data analytics (descriptive, diagnostic, predictive, prescriptive) as per your business needs.
Actionable Insights: Transform the analytical insights into actionable strategies. For instance, if analysis shows a particular product is trending, consider increasing its stock or promoting it more aggressively.
Continuous Review: The retail landscape is dynamic. Regularly review and update your analytical models to ensure they remain relevant.
Why is retail analytics so important?
Retail analytics stands as the bridge between raw data and actionable business strategies. Its importance can be encapsulated in the following points:
Customer-Centric Decisions: Through analytics, retailers can gain a deeper understanding of customer behaviors, preferences, and needs, allowing them to tailor offerings and improve customer satisfaction.
Historical Foot Traffic Analysis: By studying foot traffic patterns in various areas, retailers can identify locations that consistently attract a high volume of pedestrians, suggesting potential for robust store traffic.
Optimized Inventory Management: Retail analytics helps in predicting demand, thus preventing overstocking or stockouts – both of which can be costly.
Strategic Marketing: By understanding which marketing campaigns resonate with the audience, retailers can allocate resources more effectively, ensuring better ROI.
Competitive Edge: In a saturated market, retailers equipped with data-driven insights can identify niches, forecast trends, and make proactive decisions, giving them an edge over competitors.
Enhanced Profitability: Through price optimization, efficient inventory management, and targeted marketing, retail analytics directly contributes to improved margins and profitability.
In essence, retail analytics is the compass guiding retailers in a vast sea of data, ensuring they sail towards success and growth.
How can Retail Analytics improve the customer experience?
Retail Analytics plays a pivotal role in enhancing the customer experience, acting as a linchpin between data insights and customer satisfaction:
Personalization: By analyzing purchase histories, browsing behaviors, and feedback, retailers can tailor marketing campaigns, product recommendations, and even in-store experiences to individual customer preferences. This ensures that customers receive relevant offers and promotions, increasing the likelihood of purchases and enhancing overall satisfaction.
Streamlined Operations: Analytics can help in optimizing store layouts based on customer footfall patterns, ensuring that popular products are easily accessible. In the e-commerce space, it can guide user interface and user experience enhancements based on customer interaction data, making online shopping more intuitive.
Improved Communication: Understanding customer preferences also means knowing how and when to communicate. Retail Analytics can inform effective communication strategies, be it through email campaigns, push notifications, or other channels, ensuring customers are informed without feeling overwhelmed.
What tools and technologies are pivotal for Retail Analytics?
The landscape of Retail Analytics is buoyed by several tools and technologies, each catering to specific needs:
1. Point of Sale (POS) Systems: Modern POS systems not only handle transactions but also gather vital sales data, customer information, and inventory status.
2. Customer Relationship Management (CRM) Software: Tools like Salesforce or HubSpot capture customer interactions, purchase histories, and preferences, acting as a goldmine of customer-centric data.
3. Data Warehousing and Big Data Platforms: With the exponential growth of data, platforms like Hadoop and cloud-based solutions become essential for storing and processing vast amounts of information.
4. Data Visualization Tools: Platforms like Tableau and Microsoft Power BI allow retailers to visualize data insights, making it easier to interpret and act upon.
5. AI and Machine Learning Algorithms: Advanced analytical endeavors, especially in predictive and prescriptive analytics, leverage AI and machine learning to derive deep insights and automate decision-making processes. An innovative pioneer in leveraging AI to help retailers make decisions is Unacast Location Analytics Software.
How can small retailers benefit from Retail Analytics?
While it's easy to associate Retail Analytics with big-box retailers and expansive e-commerce platforms, small retailers stand to gain immensely as well:
1. Targeted Marketing: Even with a limited budget, analytics can guide small retailers on where to allocate their marketing resources for maximum impact, be it social media campaigns, local advertisements, or in-store promotions.
2. Inventory Efficiency: Small retailers often operate on tighter margins. Analytics can assist in optimizing stock levels, ensuring popular items are always available without tying up too much capital in overstock.
3. Understanding Localized Trends: Unlike large chains, small retailers can swiftly adapt to localized trends. Analyzing sales data can provide insights into region-specific preferences, allowing these businesses to cater effectively to their immediate community.
4. Loyalty Programs: By understanding individual customer behaviors, small retailers can devise effective loyalty programs, offering personalized rewards and incentives, ensuring repeat business.
While the scale might be different, the principles remain consistent. Even with a modest budget, implementing a basic analytical framework can offer a significant competitive edge to small retailers.