The ability to extract valuable insights from diverse data sets has become a game changer for businesses across the globe. Among the various types of data analytics available, Spatial Analytics is a significant yet often underestimated tool that can offer actionable insights through the analysis of geographic or spatial data. Understanding locations and where people are going can provide businesses with a competitive edge, allowing them to optimize operations, improve decision making, and create more targeted marketing strategies. This comprehensive guide aims to shed light on what Spatial Analytics is, its various applications across different business sectors, and how it can be leveraged for making more intelligent business decisions.
What is Spatial Analytics?
Spatial Analytics is the technique of analyzing geographic data to identify and visualize patterns, trends, and relationships within that data. Unlike traditional data analytics, which focuses primarily on the "what" and "when," spatial analytics adds an additional layer by incorporating the "where" aspect. This is important because the geographical context often provides valuable insights that can influence strategic business decisions.
For example, traditional analytics might reveal that a retail store's sales have spiked in the last quarter. While this information is useful, it doesn't tell you where most of these sales are coming from. What are the home and work areas of your shoppers? Where else do they shop? Where are they moving to and from? Spatial Analytics can dig deeper, showing you that a particular neighborhood has seen a higher volume of customers, thereby allowing for more targeted marketing or even consideration for a new store location in that area.
Significance of Spatial Analytics in the Digital Age
In today's hyperconnected world, the amount of spatial data available has increased exponentially due to the proliferation of IoT devices, smartphones, and various sensors. Whether it's tracking the movement of goods across the globe or analyzing foot traffic in a retail store, Spatial Analytics can provide a more nuanced understanding of data that traditional analytics methods may overlook.
Unacast uses GPS data and 40 other data inputs processed through its machine learning model to provide both spatial data and visual insights for businesses to perform these analyses.
Types of Spatial Analytics
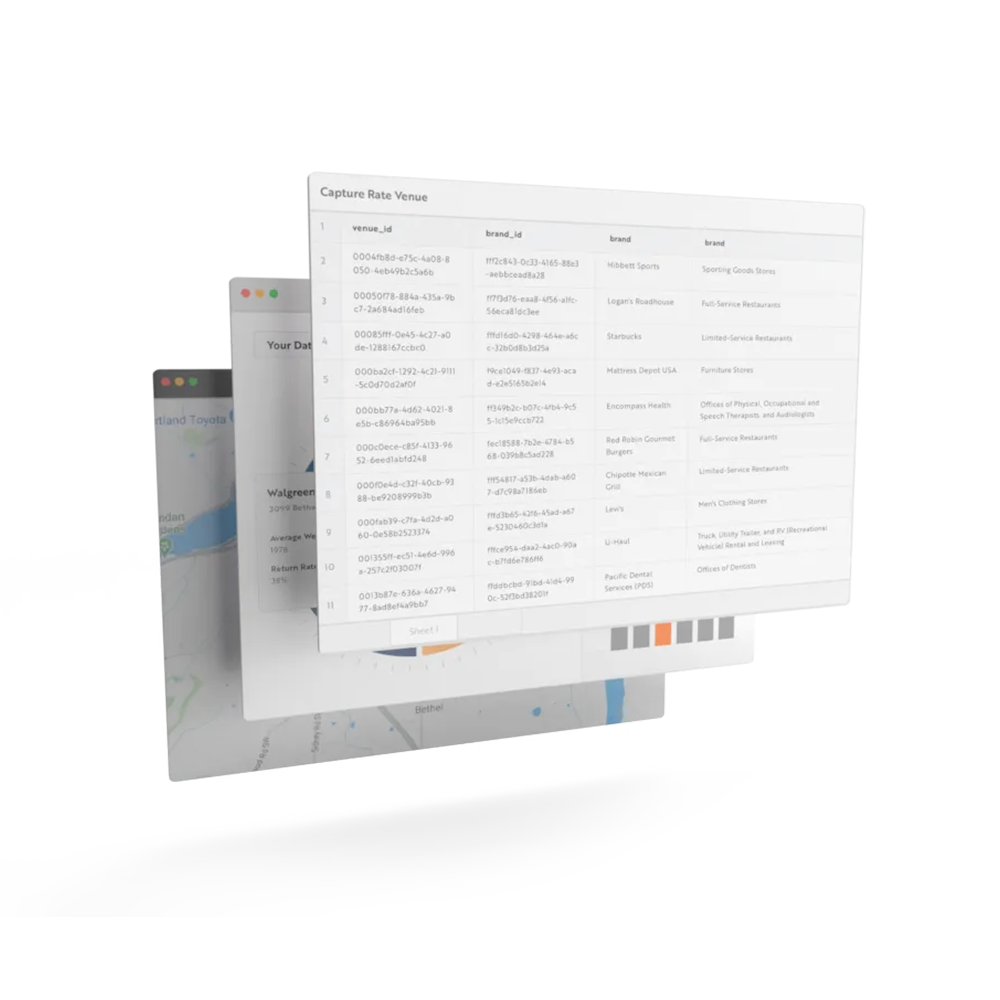
Understanding the landscape of Spatial Analytics involves recognizing the different types of analysis you can perform. Each serves a unique purpose and can provide varying levels of insight into your data. Here are the four primary types:
Descriptive Analytics
The most basic form of spatial analytics, descriptive analytics is focused on telling you what is happening or what has happened. Through the use of maps, heat maps, and other visual tools, businesses can, for example, visualize sales activity across different geographic locations. This helps organizations understand the current state of affairs in a straightforward way.
Diagnostic Analytics
This type of analysis aims to explore why something has happened. It involves a deeper look into spatial data, investigating patterns, anomalies, and correlations. For example, if a retailer observes that one store location is outperforming others, diagnostic analytics can help determine which local factors (like population density, nearby attractions, etc.) may be influencing this outcome.
Predictive Analytics
Predictive analytics seeks to forecast future events or trends based on existing data. This can involve complex models that take into account various spatial factors. For example, utility companies might use predictive analytics to anticipate the future demand for energy in different neighborhoods, enabling better capacity planning.
Prescriptive Analytics
The most advanced form of spatial analytics, prescriptive analytics offers actionable recommendations for how to handle a potential future scenario. These are based on simulations and models that consider a multitude of variables. In logistics, for instance, prescriptive analytics could offer optimal routes for delivery trucks, considering factors like traffic, road conditions, and delivery windows.
Types of Businesses that Use Spatial Analytics
The utility of Spatial Analytics is not confined to any one industry. Its versatile nature means that it can offer valuable insights to a multitude of business types. Below are some industries where Spatial Analytics is making a considerable impact:
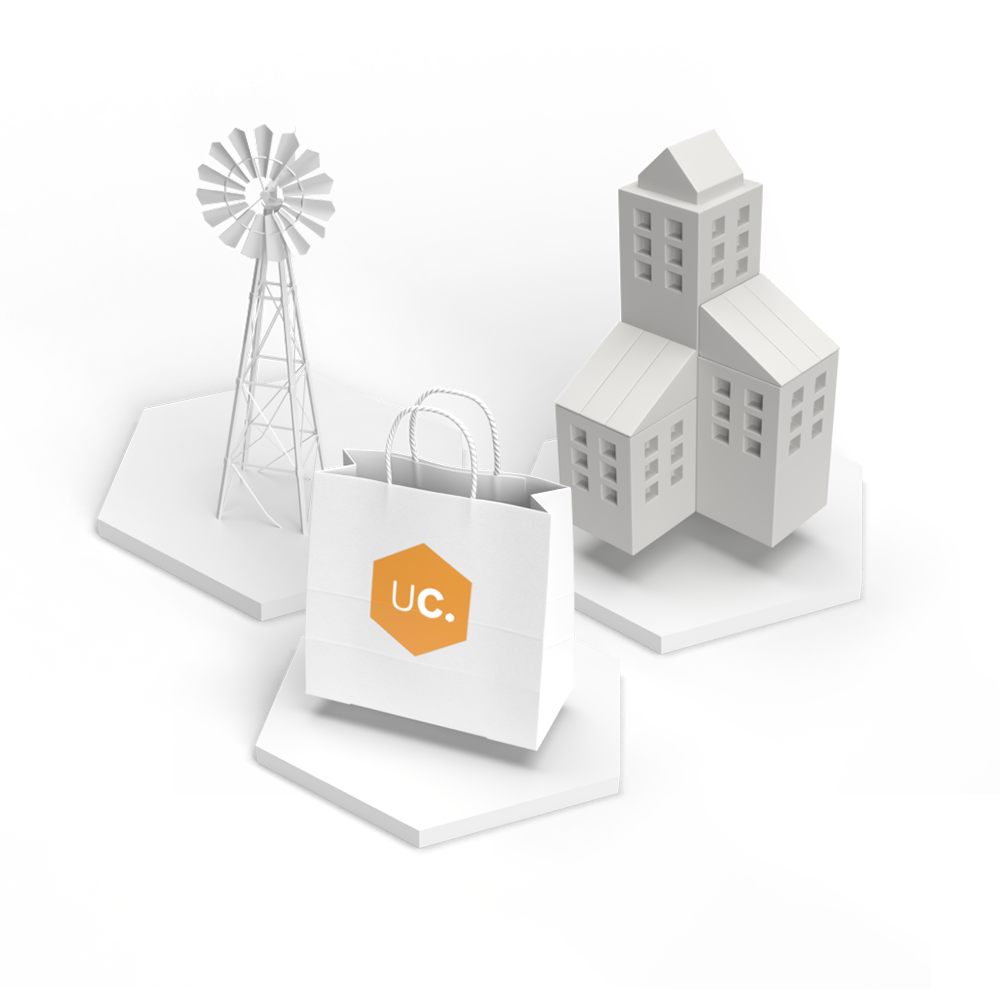
Retail and Consumer Goods
Retailers use spatial analytics to determine optimal store locations, perform competitive analysis, manage inventory based on regional demands, and design targeted marketing campaigns. With the help of spatial data, businesses can understand customer demographics on a granular level, aiding in more personalized customer experiences.
Real Estate
In real estate, the mantra "location, location, location" holds especially true. Spatial analytics can be used for site selection, market research, and assessing property value based on factors like foot traffic, changing area demographics, and consumer patterns.
Healthcare and Public Services
Spatial analytics is crucial in managing public health issues. It can be used to identify patterns in the spread of diseases or to locate areas with inadequate healthcare facilities. Government agencies can also use spatial analytics to plan public services like waste management and public transportation more efficiently.
Transportation and Logistics
In transportation, understanding spatial dynamics can help in route optimization, traffic management, and fleet maintenance. Logistics companies can significantly reduce costs and improve delivery times by analyzing and acting on spatial data.
As can be seen, Spatial Analytics can provide invaluable insights across a broad spectrum of industries, aiding in both operational efficiency and strategic planning. Whether you are a retailer looking to expand, a healthcare provider aiming to improve services, or a logistics company wanting to optimize routes, spatial analytics offers the tools you need to make more informed decisions.
With this understanding of the types of Spatial Analytics and its applications across various business sectors, you can start to see its potential for driving intelligent business decisions. In subsequent sections, we will explore how to implement these strategies practically.

Benefits of Using Spatial Analytics
Spatial analytics offers numerous advantages that can have a transformative impact on businesses. Here are some key benefits:
Improved Decision Making
Incorporating location context into your data analysis opens new dimensions of understanding. This added layer of information can significantly improve the quality and accuracy of business decisions, whether they are operational, tactical, or strategic. For instance, you can validate a potential investment based on area foot traffic and how consumer profiles are changing in your market or neighborhood.
Cost Efficiency
Spatial analytics can identify inefficiencies in business operations such as logistics, supply chain, and resource allocation. By optimizing these areas, businesses can save money and improve productivity. For instance, trains can find the most likely to be utilized routes, while retailers can allocate resources more efficiently across locations.
Competitive Advantage
Spatial insights can be a significant differentiator in today's competitive market. Understanding customer behavior on a geographical level can lead to more effective marketing strategies, better customer engagement, and an overall more targeted approach to business.
Risk Mitigation
Whether it’s identifying declining areas early, or discovering decreases in foot traffic prior to the impact on revenue, spatial analytics can help businesses anticipate and mitigate risks effectively.
Tools and Software for Spatial Analytics
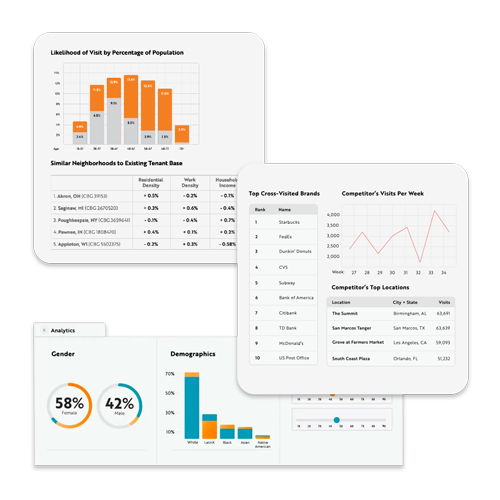
The rise of Spatial Analytics has been facilitated by the development of specialized tools and software designed to collect, analyze, and visualize spatial data. Here are some of the most commonly used platforms:
Location Insights
Unacast provides spatial analytics via its location insights platform. You can understand foot traffic to a location or area, identify consumer cross-shopping behaviors, analyze trade areas, and explore migration trends and demographic shifts in specific areas.
GIS (Geographic Information Systems)
Software like ArcGIS and QGIS allow users to create, manage, and analyze spatial data through a range of functionalities. GIS data software can handle a multitude of data types, making it versatile for different industries. Plus it can assist in things like GIS site selection and implementing GIS data management best practices.
Spatial Databases
Databases like PostgreSQL with PostGIS extensions, or Microsoft's SQL Server with spatial capabilities, help in storing and retrieving spatial data efficiently. These databases are essential for managing large datasets and conducting complex queries.
Analytics Platforms
Software like Tableau and Alteryx offer spatial analytics functionalities that can integrate with other forms of data analytics. These platforms are especially useful for businesses that require a unified analytics solution.
Data Visualization Tools
Tools like Google Maps API, Mapbox, or Leaflet allow for the visualization of spatial data, which can be embedded into reports or dashboards for easier interpretation and decision making.
Challenges and Ethical Considerations
While Spatial Analytics offers a plethora of benefits, it is essential to be aware of its challenges and ethical considerations:
Data Privacy
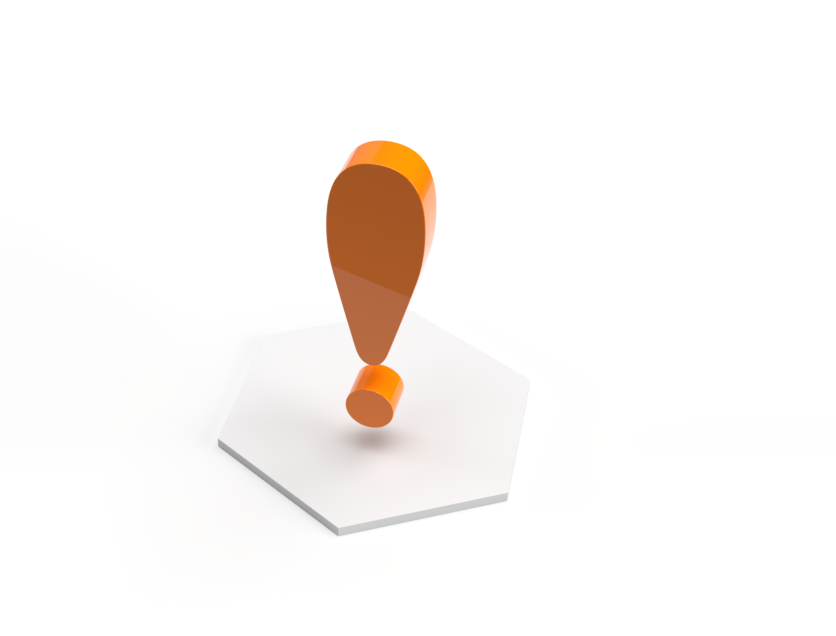
The use of spatial data can sometimes border on invasive, especially when it comes to tracking individual behaviors. Companies must adhere to privacy laws such as GDPR in Europe or CCPA in California when collecting and analyzing spatial data that could identify individuals.
Data Accuracy and Reliability
The quality of insights derived from spatial analytics is heavily dependent on the accuracy of the data being used. Inaccurate data can lead to faulty analyses, making it crucial to verify the reliability of data sources. This is why Unacast employs a rigorous methodology for maintaining its ground truth correlation of at least.93.
Complexity and Resource Requirements
Advanced forms of spatial analytics can be resource intensive, requiring specialized skills and computing power. Small businesses might find the costs prohibitive, making it essential to weigh the benefits against the resource investment needed.
This is why Unacast focuses on making it easy for teams to access both location data and insights. Our analytics platform provides generative AI summaries utilizing machine learning models to provide the industry's most accurate and easy to use location insights. To learn more, book a meeting with us.
The world of Spatial Analytics is both fascinating and complex, offering incredible opportunities for business optimization and growth. However, like any powerful tool, it comes with its own set of challenges and ethical considerations. By understanding both the capabilities and limitations of spatial analytics tools and software, businesses can make informed decisions that are not only smart but also responsible.
Future Trends and Developments in Spatial Analytics
As technology continues to evolve, so does the scope and application of Spatial Analytics. Here are some future trends that could redefine how businesses use spatial data:

Integration with Machine Learning and AI
Machine learning algorithms can analyze vast datasets much faster and more accurately than human analysts. The integration of machine learning and AI with spatial analytics leads to far more accurate predictive and prescriptive models. To learn more about our models, book time with us.
Real-Time Analytics
With the advent of IoT devices and real time data collection methods, Spatial Analytics is set to become even more dynamic. Real time analytics will allow businesses to make decisions instantly, reacting to current events or changes in patterns as they happen.
Augmented Reality (AR)
The use of AR in spatial analytics can revolutionize data visualization. Imagine walking through a future real estate development virtually, with realtime data overlay providing insights into various aspects like lighting, foot traffic, and even projected property values.
Automated Decision Making
As spatial analytics tools become more sophisticated, automated decision making systems could become more prevalent. These systems would make operational decisions based on a constant stream of incoming spatial data, with minimal human intervention required.
Conclusion
Spatial Analytics is a powerful tool that goes beyond traditional analytics by adding a geographical dimension to data. This enables businesses to make more informed decisions, improve efficiency, and gain a competitive advantage. While it offers immense possibilities, it also comes with its own set of challenges and ethical considerations that businesses need to navigate carefully.
From retailers mapping out the most profitable locations for their next store, to healthcare providers optimizing the placement of emergency services, the applications are as diverse as they are impactful. And with the continual advancements in technology, the future of Spatial Analytics looks promising, filled with ever more sophisticated tools and far-reaching applications.
Understanding the nuances of spatial data, coupled with the right analytical tools, can unlock unparalleled insights, driving businesses toward more intelligent and informed decision making. So, whether you're just starting to explore Spatial Analytics or are already well versed in its potential, the sky's the limit for what this powerful form of analytics can help you achieve.
Frequently Asked Questions on Spatial Analytics
What is an example of spatial data?
Spatial data is any information related to geographical coordinates or locations. For instance, consider the use of spatial data in a commercial real estate investment decision. An investor might compile a comprehensive dataset that includes area demographics, local shopping behavior, foot traffic, public transportation routes, and more. Spatial data is the bedrock upon which sophisticated analyses and data visualizations can be built, making it an essential tool for any field that requires a deep understanding of geographical elements. In a business context, this might involve optimizing delivery routes to save on fuel costs or identifying the best location for a new store based on population density, nearby competitors, and local amenities.
What are the different types of spatial analysis?
Spatial analysis is a broad field and can be categorized into four main types: Descriptive Analytics, Diagnostic Analytics, Predictive Analytics, and Prescriptive Analytics.
1. Descriptive Analytics:
This is the simplest form of spatial analysis, aiming to provide a snapshot of what has happened or is currently happening. It often involves creating maps or other visual representations to understand data at a glance. For example, a heat map of customer interactions across different retail locations can show which areas have the most foot traffic.
2. Diagnostic Analytics:
Going a step further, diagnostic analytics tries to dig into why certain geographical trends or patterns exist. It's useful for understanding the causeandeffect relationship between spatial elements. For instance, it can help pinpoint why a particular neighborhood has seen a spike in crime rates.
3. Predictive Analytics:
Using historical data and statistical models, predictive analytics aims to forecast future events. For businesses, this could mean predicting future sales in different locations, helping them to manage stock levels proactively.
4. Prescriptive Analytics:
This is the most advanced type, offering actionable insights based on complex models. It prescribes specific actions to achieve desired outcomes. In a logistics context, it could suggest the most efficient delivery routes that take real time traffic conditions into account.
Each type serves specific needs and provides varying levels of insight, and they are often used in combination for the most comprehensive analysis.
What is spatial analysis also called?
Spatial analysis is often interchangeably referred to as "geographic analysis" or "geospatial analysis." All these terms refer to the practice of examining the spatial relationships between different types of data. However, the terminology can vary based on the field or industry. In fields like geography or urban planning, the term "geographic analysis" is commonly used. In contrast, industries that heavily rely on technology and data science might prefer the term "geospatial analysis." This is because "geospatial" emphasizes the data's analytical and computational aspects, often involving complex algorithms and machine learning techniques. Regardless of the terminology, the core concept remains the same: analyzing data in a geographic or spatial context to gain valuable insights that can inform decision making. Whether you are looking at the spread of a disease, the effectiveness of a marketing campaign, or the best location for a new facility, the underlying principles of spatial analysis apply.